
Real-Time Personalization
Incorporating a personalized shopper experience on an automotive website using supervised learning methodologies to recommend products and provide the closet local dealerships they are available at.
Client
INFINITI USA
Location
Calgary, AB
Duration
1 Month
Project Type
Data Science
Overview
- Global development and collaboration with stakeholders located in Canada, USA and Germany
- Current set up of the automotive site is an overall encompassing national site that hopes to drive traffic to any individual dealer ship website. However, with multiple CTAs peppered throughout the site, conversion from the national site to the local sites was 0.27%.
Solution
- By incorporating real-time personalization (RTP), we curated information on anonymous website visitors and classified this data based on business demographics. We were able to serve relevant content and increase dealer website shopping.
- User experience was built into a modal pop-up that built it's content based on the user's journey. There were different combinations of model specific, body specific, generic content depending on what was available.
- Test bed was split into control, test and random groups. This way we could analyze the uplift between a data science driven model vs a rule-based model.
- We measured views, CTRs, exit rates, visits on both sites and rates of specific metrics on the dealer website.
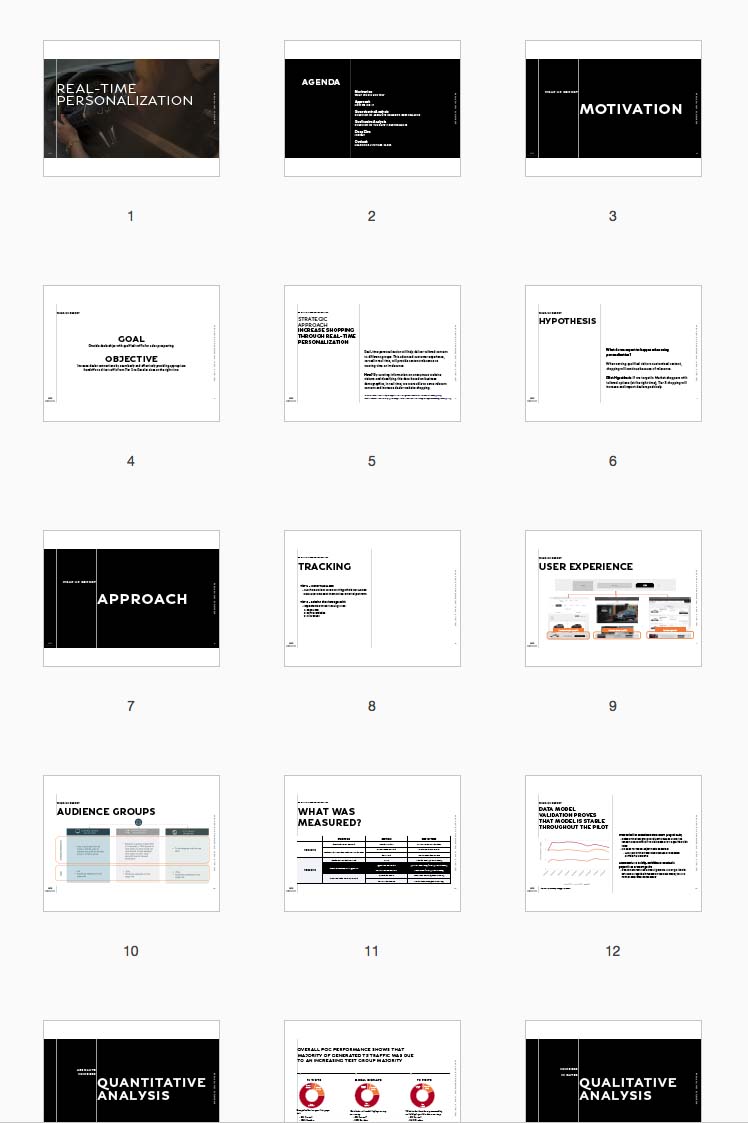
Results
After 4 weeks, the pilot has displayed some exceptionally strong performance
- ~160% dealer website visits
- ~120% dealer qualified website visits
- ~60% dealer calls
- ~50% dealer lead forms
AI driven RTP proves to be the most efficient approach to move qualitative traffic to T3 pages and increases user interaction with dealer positivity. RTP is a mechanism that can affect and adjust performance effectively and with a transparent business value